⚡ 最新课程推荐:
- 时间: 6 月 14-15;21-22;28-29 日 ;每天9:00-12:30
- 方式: 线上直播+30天回放
- 嘉宾: 徐轶青 (斯坦福大学)
- 资料: 课程主页 | PDF 课纲 | 文献
- ⛳ 点我报名 | 助教招聘
FAQ:往期课程板书和答疑文档
选题征集,点击 此处 或者扫码填写问卷留下您的宝贵意见,感谢!
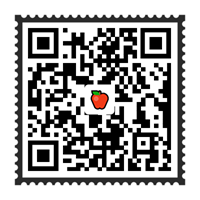
NEW!视频课堂:lianxh-class.cn
⚡ 新版 lianxh 命令:使用说明
. ssc install lianxh, replace
. lianxh 多期DID
⚡ ihelp
:帮助文件 PDF 版
. ssc install ihelp, replace
. ihelp xtreg
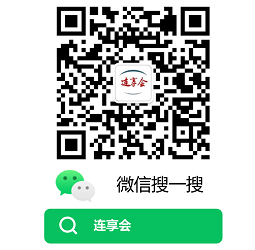